Alzheimer’s Disease Markers Found in Speech Patterns
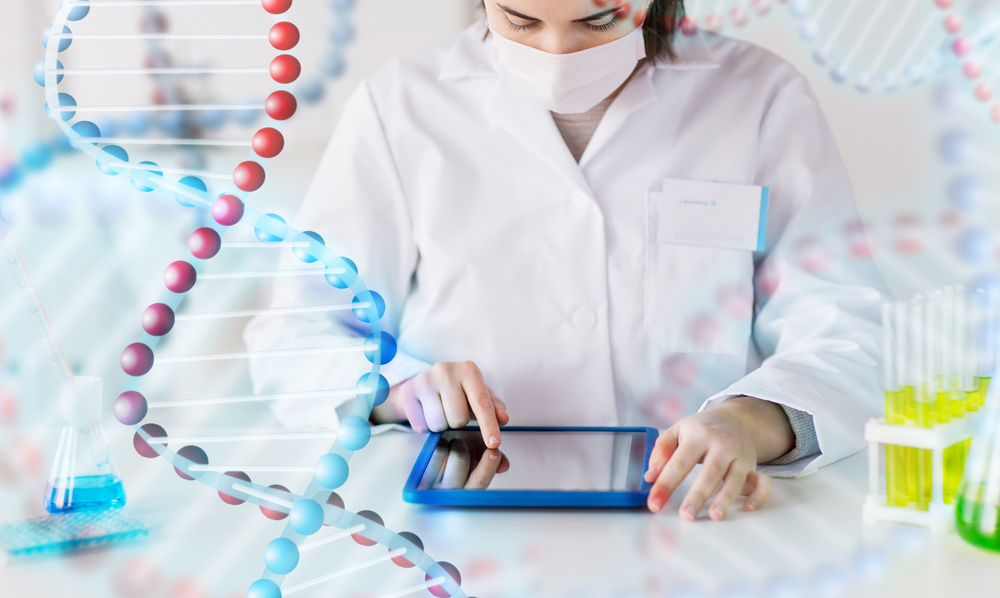
Scientists with the University Health Network (UHN) in Toronto, Canada, have discovered a method of diagnosing Alzheimer’s disease with more than 80 percent accuracy. The innovative technique evaluates the interplay between four linguistic factors, and the researchers are developing automated technology to detect these impairments.
The study, led by Dr. Frank Rudzicz, a scientist at the UHN’s Toronto Rehabilitation Institute (TR), is published in the December issue of the Journal of Alzheimer’s Disease.
The researchers report that the method and automated application of the assessment is more accurate than current Alzheimer’s assessment tools used by healthcare professionals, and can also provide an objective diagnostic rating for dementia.
In the article, titled “Linguistic Features Identify Alzheimer’s Disease in Narrative Speech” (J Alzheimers Dis. 2015 Oct 15;49(2):407-22. doi: 10.3233/JAD-150520), the researchers note that although memory impairment is the main symptom of Alzheimer’s disease (AD), language impairment can be an important marker. Relatively few studies of language in AD, however, quantify impairments in connected speech using computational techniques.
In their research, the investigators aimed to demonstrate their method’s accuracy in identifying Alzheimer’s disease from short narrative samples elicited from a picture description task, and to uncover the salient linguistic factors with a statistical factor analysis.
Based on their analysis, they determined that four collective dimensions of speech are indicative of dementia: semantic impairment, such as using overly simple words; acoustic impairment, such as speaking more slowly; syntactic impairment, such as using less complex grammar; and information impairment, such as not clearly identifying the main aspects of a picture.
Data (speech samples including audio files) were derived from the DementiaBank corpus, from which 167 patients diagnosed with “possible” or “probable” AD provide 240 narrative samples, and the 97 controls provide an additional 233. The researchers computed a number of linguistic variables from the transcripts, and acoustic variables from the associated audio files, and used these variables to train a machine learning classifier to distinguish between participants with AD and healthy controls. To examine the degree of heterogeneity of linguistic impairments in AD, they followed an exploratory factor analysis on these measures of speech and language with an oblique promax rotation, and provided interpretation for the resulting factors.
“We obtain state-of-the-art classification accuracies of over 81 percent in distinguishing individuals with AD from those without based on short samples of their language on a picture description task,” the investigators reported. “Four clear factors emerge: semantic impairment, acoustic abnormality, syntactic impairment, and information impairment.”
The authors concluded that modern machine learning and linguistic analysis will play an increasingly useful and prominent role in assessing Alzheimer’s disease.
“Previous to our study, language factors were connected to Alzheimer’s disease, but often only related to delayed memory or a person’s ability to follow instructions,” says Dr. Rudzicz, who is also an assistant professor at the University of Toronto’s Department of Computer Science and a network investigator with the AGE-WELL Network of Centres of Excellence. “This study characterizes the diversity of language impairments experienced by people with Alzheimer’s disease, and our automated detection algorithm takes this into account.”
Dr. Rudzicz adds: “The driving force that makes this analysis so accurate is the large number of measurements, behind the scenes, that are precisely and automatically detected from speech using our software. An advantage of this technology is that it is repeatable — it’s not susceptible to the sort of perceptual differences or biases that can occur between humans.”
“Every caregiver knows that people with dementia have good days and bad days — we can tell this by talking to them, because speech is a rich source of information on the brain’s cognitive function,” says study co-author Dr. Jed Meltzer, a neurorehabilitation scientist with the Rotman Research Institute at Baycrest Health Sciences, a premier international center for the study of brain function. “These methods offer a way to assess speech quantitatively and objectively, so we can use them to test interventions such as novel drugs and brain stimulation.”
“The demand on the health-care system to support Alzheimer’s disease will continue to grow rapidly,” says Dr. Rudzicz. “Our automated approach will provide an opportunity to give people easier, more cost-effective and accurate access to initial dementia screening.”
The researchers will now begin testing the automated screening technology with patients to validate the approach. Dr. Rudzicz is also partnering with the University of Toronto and industry to commercialize technology that can quickly and accurately detect signs of cognitive impairment from a sample of speech through a start-up company called WinterLight Labs. By analyzing short, one- to five-minute snippets of speech, the company’s software — which is based on years of experience and academic, peer-reviewed research — can paint a picture of the speaker’s cognitive state, including lexical diversity, syntactic complexity, semantic content, and acoustics.
The WinterLight Labs team, which includes Maria Yancheva, Kathleen Fraser, Liam Kaufman, and Dr. Rudzicz, continues to actively publish in computer science and neuroscience journals and conferences.
Sources:
University Health Network
Journal of Alzheimer’s Disease
Toronto Rehabilitation Institute
WinterLight Labs
Rotman Research Institute
Baycrest Health Sciences