Machine Deep Learning Shows Ability to Spot People Who Developed Alzheimer’s Years in Advance of Diagnosis
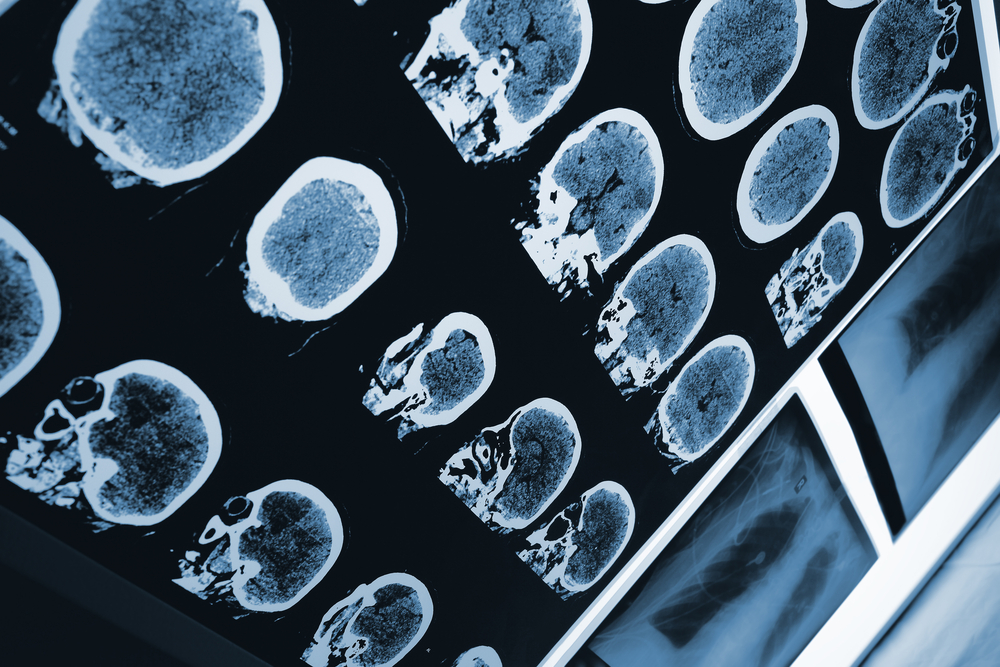
Deep learning, a type of artificial intelligence, used brain imaging scans to predict — years in advance and with nearly 100% sensitivity — patients who eventually developed Alzheimer’s disease, a study reports.
The study, “A Deep Learning Model to Predict a Diagnosis of Alzheimer Disease by Using 18F-FDG PET of the Brain,” was published in the journal Radiology.
A timely diagnosis of Alzheimer’s disease is key for better treatment and intervention, but is often challenging. Usually, changes in metabolism identified by glucose uptake in certain brain regions can provide clinical clues during the disease’s early stages. However, these changes are often difficult to identify.
Advancements in imaging diagnostic technology, such as 18-F-fluorodeoxyglucose positron emission tomography (18F-FDG-PET), can allow for earlier diagnosis and treatment.
In an 18F-FDG PET scan, FDG, a radioactive glucose compound, is injected directly into the blood. PET scans then measure the uptake of FDG in brain cells, which is an indicator of metabolic activity.
“Differences in the pattern of glucose uptake in the brain are very subtle and diffuse,” Jae Ho Sohn, MD, at the University of California in San Francisco (UCSF) and a study co-author, said in a press release. “People are good at finding specific biomarkers of disease, but metabolic changes represent a more global and subtle process.”
A continuous spectrum from normal cognition to severe impairment marks Alzheimer’s, and includes mild cognitive impairment (MCI) as a prodromal (early) stage of the disease.
Normally, Alzheimer’s patients tend to show specific patterns of metabolic activity on 18F-FDG PET scans in specific brain regions that are distinct from those with MCI. However, a substantial overlap in 18F-FDG PET scan findings are evident in both MCI and Alzheimer’s patients.
As such, 18F-FDG PET interpretation is particularly challenging when attempting to distinguish between a normal brain, and brains of MCI and Alzheimer’s patients.
Studies have shown that deep learning can help address the increasing complexity and volume of imaging data. Deep learning is a type of artificial intelligence in which machines learn by example, much like humans do.
Work is underway to apply deep learning in many diseases and imaging methods, such as breast cancer detection with mammography. But the method has not yet been integrated into clinical flow to be developed and validated.
Researchers wanted to understand whether a deep learning algorithm could be trained to predict the final clinical diagnoses of patients who underwent brain 18F-FDG PET scans and found changes in brain metabolism predictive of Alzheimer’s disease.
They used data from the Alzheimer’s Disease Neuroimaging Initiative (ADNI), a major multi-site study focused on clinical trials to improve disease prevention and treatment.
The ADNI dataset consisted of more than 2,100 FDG-PET brain images from 1,002 patients.
The researchers trained the deep learning algorithm using 90% of the dataset, and then tested it on the 10% that remained. Through deep learning, the algorithm was able to teach itself to identify metabolic patterns that were specific to Alzheimer’s disease.
They also tested the algorithm on a different set of 40 imaging scans from 40 patients who were not enrolled in ADNI and that the algorithm had never studied (called an independent test set).
The algorithm had the ability to distinguish patients who progressed to Alzheimer’s at the time of imaging (92% in ADNI test set and 98% in the independent test set) from those who would remain with MCI or non-Alzheimers/MCI (73% in ADNI test set and 84% in the independent test set). However, it was weaker at discriminating people who went on to develop MCI (63% in ADNI test set and 52% in the independent test set).
“With a perfect sensitivity rate and reasonable specificity on AD [Alzheimer’s disease], the model preserves a strong ability to predict the final diagnoses prior to the full follow-up period that, on average, concluded 76 months [about six years] later,” the researchers wrote.
Additionally, the algorithm outperformed three radiology readers (imaging experts) with statistical significance in predicting a final Alzheimer’s diagnosis in people in the independent test set.
“We were very pleased with the algorithm’s performance,” Sohn said. “It was able to predict every single case that advanced to Alzheimer’s disease.”
Still, he added that the test set was small and the algorithm needs further validation in a larger multi-institutional study. But if validated, it can prove very useful in identifying Alzheimer’s early on, when therapies might be more effective.
“If we diagnose Alzheimer’s disease when all the symptoms have manifested, the brain volume loss is so significant that it’s too late to intervene,” Sohn said. “If we can detect it earlier, that’s an opportunity for investigators to potentially find better ways to slow down or even halt the disease process.”