Simple Language Test May Predict Alzheimer’s Onset Years Before Symptoms Start
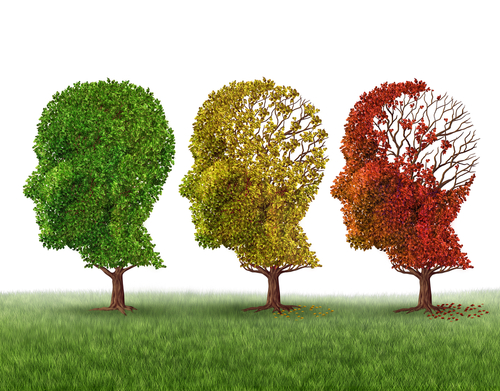
A simple language test, when combined with an artificial intelligence model, can predict which people will develop Alzheimer’s disease later in life, a study shows.
This new model predicted Alzheimer’s seven years before its diagnosis with an accuracy of 70%, according to the study. That rate is higher than those attained by traditional predictive methods, such as neuropsychological testing, the researchers said.
These findings may help in the development of simple, non-invasive, and inexpensive language tests to help clinicians assess a person’s risk of developing Alzheimer’s. Such assessments, in turn, can lead to earlier interventions, the investigators noted.
The study, “Linguistic markers predict onset of Alzheimer’s disease,” was published in the journal The Lancet eClinicalMedicine.
Increasing efforts are being made to develop easier, low-cost, reliable biomarkers of pre-symptomatic Alzheimer’s that allow clinicians to predict whether and when a person will develop the disease.
While there is still no effective cure or preventive treatment for Alzheimer’s, the earlier clinicians can detect it, the more likely they will potentially be able to delay and treat its progression.
“Aging-related cognitive decline manifests itself in almost all aspects of language comprehension and production,” the researchers wrote, noting that “even seemingly mundane linguistic abilities, such as object naming, engage extensive brain networks.”
As such, linguistic performance is a sensitive indicator of cognitive impairment and has the potential to be used to predict Alzheimer’s. In agreement, previous studies and case reports have provided evidence of an association between linguistic abilities and the transition to Alzheimer’s later in life.
Now, researchers at Pfizer and the IBM Thomas J. Watson Research Center, in New York, developed a linguistic performance-based, machine-learning model that can predict, with a high level of accuracy, the onset of Alzheimer’s years before its first symptoms manifest in cognitively normal people.
Machine-learning is a form of artificial intelligence that uses algorithms to analyze data, learn from its analyses, and then make a prediction about something.
To build the model, the team used data culled from the participants of the ongoing Framingham Heart Study (FHS). Launched in 1948, the FHS is a community-based, multi-generational, longitudinal study that enrolled more than 9,000 people to investigate various aspects of the participants’ health, particularly cardiovascular disease.
FHS participants frequently complete a battery of neuropsychological assessments, which includes the cookie-theft picture description task (CTT) from the Boston Aphasia Diagnostic Examination. This language test is commonly used for diagnosing dementia and other cognitive diseases.
In the test, participants are asked to write down what they see in a picture that includes three people in a kitchen: a woman wiping a dish at an overflowing sink, a boy reaching from atop a teetering stool into a cookie jar in the closet, and a girl with her arm outstretched expecting to get a cookie from the boy.
Use of this test could potentially provide linguistic markers to predict Alzheimer’s.
For the analysis, participants who developed mild cognitive impairment — later diagnosed as Alzheimer’s — at or before reaching age 85 were classified as Alzheimer’s cases, while those who remained dementia-free or developed the disease after age 85 were identified as controls.
“According to this definition, all cases have already developed cognitive impairment due to AD [Alzheimer’s disease] at 85, and none of the controls have developed cognitive impairment due to AD at 85,” the researchers wrote.
A total of 195 participants (63 cases and 132 controls) with dementia status derived only from the neuropsychological test-battery were used for machine learning training.
The researchers extracted 87 linguistic variables from the patients’ CTT responses and used them to train the model to detect subtle language changes, including grammatical errors and different sentence structure, and associate them — or not — with the future development of Alzheimer’s.
The model was then tested in a group of 80 participants, which included 40 cases and 40 age-, gender-, and education-matched controls, whose data had been reviewed for cognitive decline and dementia status by a panel of at least one neurologist and one neuropsychologist.
In the test group, the mean time from cognitive normality to cognitive impairment was 3.93 years and to mild Alzheimer’s diagnosis was 7.59 years. A single CTT response from each participant before any signs of mild cognitive impairment was used for the analysis.
The results showed the new language-based model could predict with 70% accuracy if a person would develop Alzheimer’s, which was significantly better than the 59% accuracy of standard prediction methods based on a person’s demographic and clinical data.
The future occurrence of Alzheimer’s also was found to be associated with repetitiveness, misspellings, and telegraphic language, which is defined as language that lacks fluent grammatical structure and continuity.
These variables “have been consistently identified in the literature as associated with cognitive decline in dementia,” the researchers wrote.
In addition, the model was found to predict the onset of Alzheimer’s more accurately in participants who did not have a college education and in women. This is consistent with data suggesting that higher education can lead to a greater “cognitive reserve” — higher resistance to brain disease — and with the fact that Alzheimer’s is more common and progresses faster in women than in men, the team noted.
“The results suggest that language performance in [natural language tests] expose subtle early signs of progression to AD in advance of clinical diagnosis of impairment,” the researchers wrote.
Compared with previous research on predicting the future onset of Alzheimer’s, this study has several advantages, the researchers said. Among them were the use of a single response to a relatively simple and natural language test when people were cognitively healthy and had no other disease risk factors.
“Simple, naturalistic and inexpensive speech [tests], as our results suggest, can provide an assistive tool for the early detection and progression monitoring of [Alzheimer’s disease], particularly given that such [tests] can be easily adapted to remote digital platforms with low patient burden,” the team concluded.
This type of tool also could help identify people who could benefit from participating in clinical trials evaluating potential preventive therapies for Alzheimer’s, the team said.
Future studies involving geographically and racially diverse populations are needed to confirm the predictive value of this language test with AI in the general population.